After studying theoretical physics, Florian Hartig was a research assistant at the University of Freiburg and since 2016 he has been a W2 professor at the University of Regensburg.
His research focuses on theory, simulation models and statistical methods in ecology, evolution and nature conservation. Examples of newer activities are the development of statistical software (e.g. the R-packages DHARMa and BayesianTools), the integration of model data of dynamic vegetation models (e.g. Hartig, F., Dyke, J., Hickler, T., Higgins, S., O’Hara, R., Scheiter, S. & Huth, A. (2012): Connecting dynamic vegetation models to data – an inverse perspective. J. Biogeogr., 39, 2240-2252. Online version), statistical applications, method comparisons and the coexistence theory.
In addition to browsing his publications (e.g. on Google Scholar), you can find out more about his recent activities on his research blog, on GitHub or on Twitter: @florianhartig
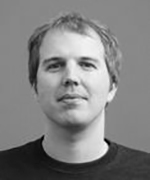
Theoretical ecology
I represent the subject “Theoretical Ecology”. In my working group we use statistical methods and simulations to develop theories about the organizational principles of ecosystems and to compare them with data. An important field of research for us is the reaction of ecosystems, especially forest ecosystems, to climate change. The latter is also the main topic of the junior research group of Dr. Hülsmann, which is financed by the Bavarian climate research network bayklif and associated to my group. But we also do research on other topics, in particular the formation and conservation of regional and global biodiversity patterns, species interactions, and much more.
It depends on the course. In general, it is the ability to understand statistical and AI problems and to be able to solve them practically. The exact learning objective is usually described in the course catalog.
At the moment, AI or ML is simply a rapidly developing field of research with broad areas of application in research and business. The topic is almost universally relevant, from the humanities (keyword digital humanities) to the life sciences and the “hard” natural sciences.
As I said, we work at the interface between ecological theory and data. This means that we evaluate (large) ecological data and program computer simulations, but we also develop statistical software and methods (see here).
I originally studied physics and have been working in the field of modeling / software / statistics since my doctoral thesis.
It depends on what you mean by “find it easy or difficult”. The AI / ML area is much younger and less developed than, for example, the field of statistics. That tends to make research easier and more creative. It is also the case that in the field of AI there is currently a mood that resembles a “gold rush”, both with regard to the funding provided and with regard to the interest of scientific journals etc. Due to these positive external conditions, the field is also moving at an insane speed, so you have to stay on the ball, and there is a significant risk of other people working on the same problem.
What interests me in statistics as a whole is the challenge of building and understanding algorithms that generate answers about the essence of nature from data. Whether it’s a regression or a deep neural network doesn’t really make that big a difference to me.
Not in teaching, but we have various interdisciplinary collaborations in research. The website of my working group or my list of publications give an overview.
I see AI initially not as a subject, but an application that brings together more basic disciplines. The development of AI applications requires the corresponding specialist knowledge, which typically comes from the fields of statistics, computer science, mathematics and the respective departments for which AI applications are to be developed. I think it is therefore important to develop the necessary basic skills for AI first in the traditional disciplines of the university, but also in school. By this I mean primarily mathematics, statistics and programming, which should be strengthened both in school education and in the university curricula. Building on those, we can develop more specialized AI courses and I think at the same time some basic knowledge about AI should actually be anchored also in every MSc program, including the humanities. After all, with the appropriate frameworks, building a neural network is not rocket science. In principle, you can teach that to any MSc student, and I think such a basic understanding of what AI is would be useful in the future.
I think this is an excellent initiative. Incidentally, it emerged from a commission on data science at the UR, in which I was also represented
No, I think we have everything covered, thanks for the questions!
Mr. Hartig, thank you very much for taking the time to answer these questions. We wish you a nice day!